Table Of Content
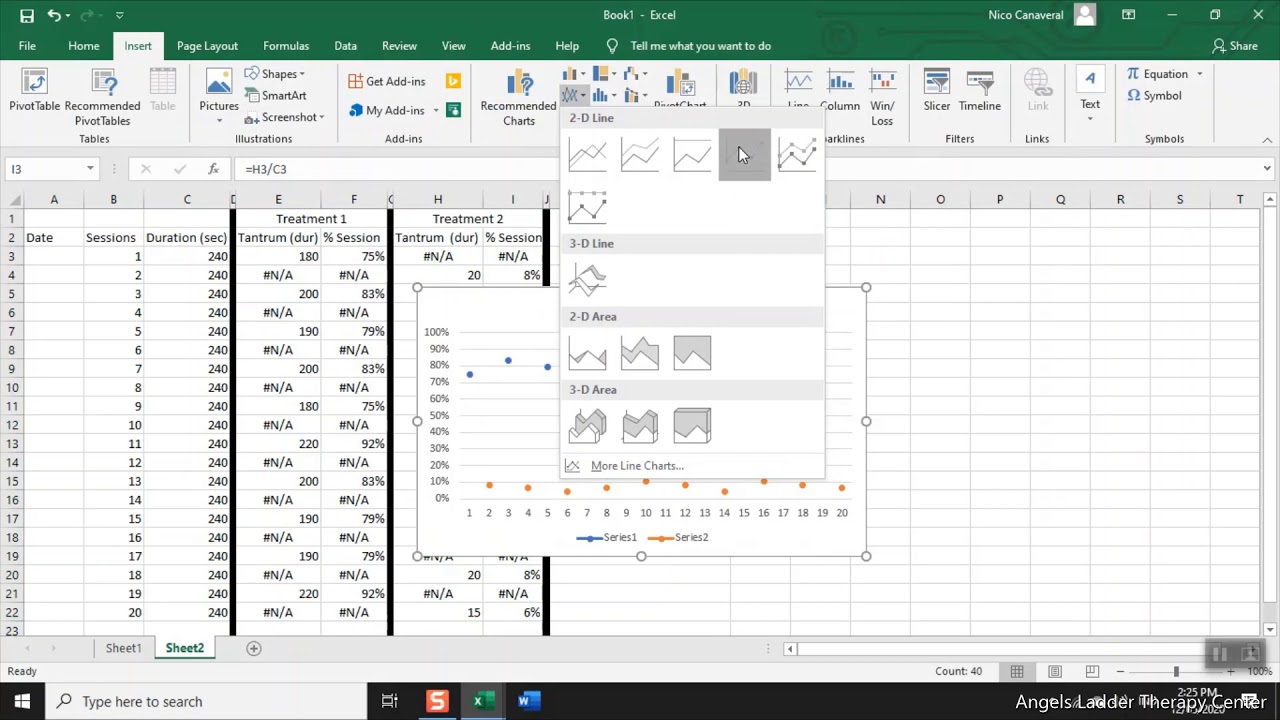
However, rather than having multiple baselines across participants, settings, or behaviors, the changing-criterion design uses multiple levels of the independent variable. Experimental control is demonstrated when the behavior changes repeatedly to meet the new criterion (i.e., level of the independent variable). In some cases, the simultaneous and continuous data collection in all legs of multiple-baseline designs is not feasible or necessary. Multiple-probe designs are a common variation on multiple baselines in which continuous baseline assessment is replaced by intermittent probes to document performance in each of the conditions during baseline. Probes reduce the burden of data collection because they remove the need for continuous collection in all phases simultaneously (see Horner & Baer, 1978, for a full description of multiple-probe designs). Pre-intervention probes in Condition 1 are obtained continuously until a stable pattern of performance is established.
A special latin square for the use of each subject “as his own control”
Moreover, the use of randomization makes possible and valid the use of randomization tests, a kind of statistical test that makes no distributional assumptions and no assumptions about random sampling (Edgington & Onghena, 2007; Levin et al., 2019). The evidence provided by the application of a randomization test to an individual’s data is more closely related to the typical aims in behavioral sciences (Craig & Fisher, 2019). Applied researchers need to be cautious only when performing multiple statistical tests, in relation to potentially committing a Type I error. Finally, statistical inference can be expressed as a confidence interval constructed around an effect size estimate, thanks to inverting the randomization (Michiels et al., 2017). A different comparison can be performed, comparing data paths, rather than only actually obtained measurements, using ALIV (Manolov & Onghena, 2018) and the visual structured criterion (Lanovaz et al., 2019).
Multiple-Treatment Designs
Multiple-probe designs may not be appropriate for behaviors with significant variability because the intermittent probes may not provide sufficient data to demonstrate a functional relationship. If a stable pattern of responding is not clear during the baseline phase with probes, the continuous assessment of a multiple-baseline format may be necessary. Incorporating randomization in the design boosts internal validity and scientific credibility in any type of design, including SCEDs (Edgington, 1975; Kratochwill & Levin, 2010).
Randomization tests for restricted alternating treatments designs.
In both cases, the consistency of effects can be conceptualized as the degree to which variability of the effects observed in the different blocks are comparable to the average of these effects across blocks. These separate assessments are well-aligned with the recommendations for performing visual analysis (Lane et al., 2017; Ledford et al., 2019; Maggin et al., 2018). For James and Joseph, the procedure resulted in target responses that also produced low baseline levels. For Sean, after completing the target identification phase and beginning baseline, the interventionist was informed that the classroom teacher independently chose to teach some of the selected responses.
About this article
Simulating a brain-cooling treatment that could one day ease epilepsy - EurekAlert
Simulating a brain-cooling treatment that could one day ease epilepsy.
Posted: Thu, 05 Oct 2017 07:00:00 GMT [source]
This notion has a relatively long history (Edgington, 1975) and continues to be mentioned in contemporary texts (Todman & Dugard, 2001). One disadvantage of all designs that involve two or more interventions or independent variables is the potential for multiple-treatment interference. This occurs when the same participant receives two or more treatments whose effects may not be independent.
Nonparametric statistical tests for single-case systematic and randomized ABAB…AB and alternating treatment ... - ScienceDirect.com
Nonparametric statistical tests for single-case systematic and randomized ABAB…AB and alternating treatment ....
Posted: Wed, 27 Dec 2017 00:58:04 GMT [source]
Direct replication refers to the application of an intervention to new participants under exactly, or nearly exactly, the same conditions as those included in the original study. This type of replication allows the researcher or clinician to determine whether the findings of the initial study were specific to the participant(s) who were involved. Systematic replication involves the repetition of the investigation while systematically varying one or more aspects of the original study. This might include applying the intervention to participants with more heterogeneous characteristics, conducting the intervention in a different setting with different dependent variables, and so forth.
Multiple-Baseline Designs
As we have seen throughout the book, group research involves combining data across participants. Group data are described using statistics such as means, standard deviations, Pearson’s r, and so on to detect general patterns. Finally, inferential statistics are used to help decide whether the result for the sample is likely to generalize to the population. Single-subject research, by contrast, relies heavily on a very different approach called visual inspection. This means plotting individual participants’ data as shown throughout this chapter, looking carefully at those data, and making judgments about whether and to what extent the independent variable had an effect on the dependent variable. There are two potential problems with the reversal design—both of which have to do with the removal of the treatment.
About
In addition, there is no known sampling distribution, making it impossible to derive a confidence interval (CI). CIs are important because they help create an interpretive context for the dependability of the effect by providing upper and lower bounds for the estimate. There has been a small but steady body of work addressing effect size calculation and interpretation for SSEDs. Space precludes an exhaustive review of all the metrics (for comprehensive reviews, see Parker & Hagan-Burke, 2007, and related papers from this group). There are, however, a number of points that can be made regarding the use (derivation, interpretation) of effect size indices that are common to all. The simplest and most common effect size metric is the percentage of nonoverlapping data (PND; Scruggs, Mastropieri, & Casto, 1987).

Traditional nonparametric approaches have been advocated, but they do not necessarily avoid the autocorrelation problem and, depending on the size of the data array, there are power issues. Alternatively, if single-subject data are regarded as time-series data, there have been some novel applications of bootstrapping methodologies relying on using the data set itself along with resampling approaches to determine exact probabilities rather than probability estimates (Wilcox, 2001). In the end, effect detection is determined by data patterns in relation to the phases of the experimental design.
If we don’t believe in unlikely events then our conclusion is tentatively that the intervention is effective, but a statistically significant result does not show the actual probability that the intervention is superior to another treatment or baseline. The alternating treatments design which is increasingly being used in single subject research is described. This design allows the differential effectiveness of two or more treatments to be investigated by presenting each of them separately in closely spaced sessions, with the order of presentation alternated. Consideration is given to the types of behavior and contexts that are most appropriate for use with this design and also to factors important for ensuring its effectiveness and the valid interpretation of the resulting data. This study replicated Seaver and Bourret (2014)’s approach to identifying effective prompt topographies prior to comparing prompt hierarchies and extended the work by providing additional support with younger populations and an alternative procedure for conducting the prompt topography assessment. The dependent variable was the percentage of correct independent responses to one-step directions.
From the perspective of scientific significance, one can argue that statistical analysis may be warranted as a judgment aid for determining whether there were any effects, regardless of size, because knowing this would help determine whether to continue investigating the variable (i.e., intervention). If it is decided that, under some circumstances, it is scientifically sensible to use statistical analyses (e.g., t tests, analyses of variance [ANOVAs], etc.) as judgment aids for effect detection within single case data sets, the next question is a very practical one—can we? In this context, the term safely refers to whether the outcome variables are sufficiently robust that they withstand violating the assumptions underlying the statistical test. The short answer seems to be “no,” with the qualifier “under almost all circumstances.” The key limitation and common criticism of generating statistics based on single-subject data is auto-correlation (any given data point is dependent or interacts with the data point preceding it). Because each data point is generated by the same person, the data points are not independent of one another (violating a core assumption of statistical analysis—technically, that the error terms are not independent of one another). Thus, performance represented in each data point may likely be influencing the next (Todman & Dugard, 2001).
No comments:
Post a Comment